Interview: Peter Hafez, Chief Data Scientist, RavenPack
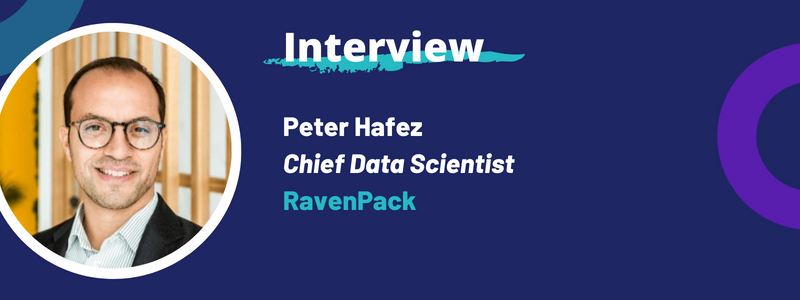
Peter Hafez is the head of data science at RavenPack. Since joining RavenPack in 2008, he’s been a pioneer in the field of applied news analytics bringing alternative data insights to the world’s top banks and hedge funds. Peter has more than 15 years of experience in quantitative finance with companies such as Standard & Poor's, Credit Suisse First Boston, and Saxo Bank. He holds a Master's degree in Quantitative Finance from Sir John Cass Business School along with an undergraduate degree in Economics from Copenhagen University. Peter is a recognized speaker at quant finance conferences on alternative data and AI, and has given lectures at some of the world’s top academic institutions including London Business School, Courant Institute of Mathematics at NYU, and Imperial College London.
Don't miss new reports! Sign up for Quant Strats Newsletter
Aminah Hanif (Content Lead, Quant Strats): Hi Peter, thank you for speaking with me today. I was hoping we could start with you giving us a little introduction on your role and what you do. Then, perhaps a bit of insight into your biggest professional achievement to date?
Peter: As the Chief Data Scientist at RavenPack, I bridge the gap between data science, product, and sales.
With 20+ years of experience in quantitative research, data science strategy, and product development from fin-tech startups to global banking, I lead distributed, mission-driven data science teams that create actionable insights from unstructured data - using nlp-driven analytics. My mission is to innovate, to create, and to communicate value-adding insights to our clients - across fundamental and quantitative mandates, across multiple asset classes and investment horizons, across various industries and geographies.
I've had the privilege of speaking at quant finance conferences on alternative data and machine learning across the globe - hosted by some of the largest financial firms in the business including Bank of America, JP Morgan, BNP Paribas, Deutsche Bank, Credit Suisse etc.. I've also enjoyed giving guest lectures at the world’s top academic institutions such as the Courant Institute of Mathematics, Stern Business School, Columbia Business School, London Business School and Imperial College London amongst others.
Being a pioneer in the Alternative Data revolution, RavenPack has had a significant impact on the financial industry over the last 20 years. In particular, we have made strong contributions to defining and creating the standard for News Analytics in finance. Today, RavenPack is seen as the market leader in the industry of nlp-driven analytics. Not only due to our long presence in the industry with proven products and research, but also since we have shown that we are capable of delivering continuous innovation and value to our clients.
Aminah: Thank you, then going further into RavenPack. What has been your /your firm’s top 3 priorities for the coming year? What are you hearing from your clients as their main priorities?
Peter: Last year RavenPack delivered EDGE – our most advanced language AI platform yet, which uses a 12-million entities knowledge graph to map the data world across news, voice transcripts, filings, and more. Our priorities this year are to leverage this platform to (1) uncover new sources of alpha in existing datasets by combining signal components, particularly around earnings season, in our major research cycle on earnings intelligence. (2) organise information in EDGE to address the business needs of investors with innovative solutions, like our ESG controversy scoring framework, (3) onboard new datasets into EDGE to open new chapters in alt data, like the complete analysis of millions of jobs descriptions. (4) Extend the relevance of alternative data to new strategies and asset classes, with macro models that deliver inflation or GDP nowcasting from language AI, and thematic strategies to underpin innovative indexes and ETFs. These directions are very well aligned with our clients’ expectations and needs.
Aminah: What do you think are the biggest challenges facing data scientists/AI experts/quantitative practitioners for 2022 and beyond?
Peter: The first challenge data scientists face is attention span. With the multiplication of datasets, models, and even the pace of innovation in the NLP space, what to focus on, and who to work with, is harder to discern. The second challenge is that data science is becoming an ecosystem function: there’s a legal aspect to data, with more prevalent emphasis on rights protection, a distribution aspect, with more platforms and marketplaces available to disseminate datasets. So quant teams have to juggle more angles beyond whether data uncovers alpha. The third challenge is the timeliness of data research: when events like the invasion of Ukraine emerge on the global geopolitical landscape, quants need to be able to adapt their models effectively in a matter of days, not months. Anticipating the varying ways that data scientists will need to access data, and how to react effectively to changing circumstances, becomes a primary driver for quants. Leading data vendors are seen more as data partners that help them shape the way they approach, maintain, and operate their data lake, as in the recent surge of interest for data mosaics.
Aminah: The devastating situation in Ukraine has also had a huge impact on quant trading – what has this been and what are your predictions? What other geopolitical shifts are happening and how are they affecting quant investing?
Peter: The Russian invasion of Ukraine has shown that trading models were too focused on isolated financial risks and failed to capture the whole picture. A more holistic approach to risk management was warranted. That new paradigm must encompass supply chain, workforce, geopolitical, distribution and reputational risks as a mosaic of interconnected influences. For instance, you cannot analyse supply chain risk without a geopolitical dimension. If Taiwan’s autonomy is challenged by mainland China, is it a supply risk for companies with suppliers in Taiwan, or a financial one for companies impacted by possible trade sanctions in the region? An effective data strategy should not require a data team to choose how they will approach an emerging problem: it should provide differentiated options that can be explored in parallel.
Aminah: To what extent do you see the use of blockchain/crypto integrating into capital markets? As crypto is becoming more mainstream, how have hedge funds responded and what could be the potential impact on capital markets?
Peter: For a long time, financial institutions were not too fascinated by crypto currencies: the way they are transacted, the fiscal and legal question-marks, the erratic volatility and the lack of nominal accountability weighed in on their appetite for crypto instruments. Quants look for signals that can be properly backtested with predictable results to maximise the likelihood of subsequent profit. By all metrics, crypto currencies could not be components of such signals. Today we observe a less timid approach towards cryptos, but few patterns have emerged, and exploring the signals that seem to influence the pricing of crypto currencies remains challenging. Whether cryptos become a reliable pillar of portfolio investment strategies remains to be seen. However, in the last 6-12 months, we have observed that the space is becoming increasingly institutionalised - with more and more experienced investors, from the hedge fund industry, launching new crypto-focused strategies. This will necessarily increase the demand for data insights on crypto assets to power better price predictions.
Aminah: ESG and sustainable investing is still a large topic, with many funds listening to investors’ demands and influencing their portfolio management. How do you see this progressing in the coming years? How are funds partnering with companies in this space to remain compliant and also get a return on their investments?
Peter: The first challenge of ESG investing is measuring ESG in the context of the activities of a company. The second challenge is to do it in a timely manner. To a large extent, investors are currently failing both, but not for long. One of the drivers of ESG today remains the detection of negative news – scandals and anomalies that deteriorate the company’s sustainability profile. For a long time investors would rely on analyst ratings that can lag scandals by several months or quarters, but such a delay is no longer acceptable for sustainability-conscious investors. These can greatly benefit from early indicators that help portfolio managers anticipate emerging risk as it unfolds. This is the main driver that brings investors to work with us on early detection, classification, and reporting based on media reports – a low-latency signal that can quickly identify emerging threats.
Aminah: Privacy and regulation surrounding the responsibility and ownership of data is still an area being discussed. What measures are you predicting will be put in place to navigate any foreseeable data privacy challenges while searching for alpha, and how can funds learn to navigate these regulations and policies? What are solutions providers doing to work around this?
Peter: Data governance is a complicated subject for any vendor who did not embed rights management and sourcing compliance into their business model. At RavenPack, we have clear agreements with reputable data providers like Dow Jones or FactSet covering news, regulatory filings, earnings call transcripts and more sources of alternative data. Other providers, particularly if they deal with personally identifiable information, have a much higher bar to clear to ensure that the information they provide is not only authorised, but also secure, that the signals they draw can be produced for the foreseeable future, and that the quality of the feed is maintained. Vendors should demonstrate their command of these topics as a first-level citizen of their value proposition, rather than promote only alpha-generative aspects of their offering. The concern funds face is whether emerging startups in this space are fully equipped to provide the robust partnership that funds need to navigate this fast-moving landscape.
Aminah: Alternative data is still considered a source of alpha for many – what roadblocks do firms tend to come across in sourcing, cleaning, and using this data? How do you view the alt data market at present and where is it heading? How can we streamline this process and is that possible?
Peter: It’s useful to examine why alternative data remains a source of alpha. Although the question may benefit from an extended answer, the salient reason is that a lot of the alpha hidden in alternative data remains untapped, so finding more alpha is less about finding new exotic sources of alpha, and more about refining and reworking existing models. Take news for instance, RavenPack’s sentiment models have consistently delivered additional alpha, but we now see three phenomena emerging: first, we are finding thematic indicators that fine-tune our signal components in specific contexts so various tilts applied to classic models uncover additional alpha in the same dataset. Second, we are now combining orthogonal signal components from multiple datasets, and finding that the result performs better than the sum of its parts. For instance, adding insider transaction data to earnings news data improves information ratios. The same goes with earnings date changes, or transcript signals. Third, we are seeing alt data contribute alpha in trading styles and asset classes we had not considered before. Take earnings call transcripts for example: we have been able to create a macro signal component from the analysis of executive presentations that improves macro strategies. Now all this is made possible by the unified knowledge graph and data model that RavenPack provides, which seamlessly integrates the various sources of alt data, so investments firms do not have to solve the hurdles of data sourcing and cleaning the data; instead they can focus on readily onboarding these signal components into their own internal models.
Aminah: And lastly, Our research this year showed a lot more firms and practitioners talking about NLP than usual – why do you think this is? Where are you seeing the optimal utility for NLP and where does it have the potential to go?
Peter: A simplistic answer would be that textual data continues to grow, and 80% of all new data is unstructured, so the prominence of NLP comes with the need to tap those data sources, but that would leave out the key aspect. The investment industry is at a turning point where powerful language AI models are now driving innovation. In areas of investment focus, like ESG, language AI not only analyses data to uncover alpha, but it is instrumental to the understanding of the fundamentals of the topic. How do you detect greenwashing? How do you identify which companies are having a positive impact on sustainability? This shift requires a combination of traditional heuristic algorithms and machine learning models to move from detecting to defining, and to move from individual signals to knowledge graphs. NLP is becoming the operating system that underpins both human intelligence and signal intelligence analytic processes, and that’s why it’s becoming more prominent.
Aminah: That's great, it's nice to hear about what RavenPack has been working on and the challenges the industry will be facing in this following year. Thank you for talking with me today!
Peter: It's been lovely to chat with you Aminah, thank you for having me.
Don't miss Peter Hafez at Quant Strats Europe 2022, LIVE and in-peron at the Park Plaza Victoria, London with 40+ top experts and 200+ attendees, and learn more about the quantitative data industry. Join Peter Hafez for the KEYNOTE PANEL: Exploring the value in previously under-utilised assets to maximize your profit, with the panelists Igor Yelnik, CIO and CEO, Alphidence Capital; Rob Huisman, Quantitative Researcher, Robeco; Xander Saveberg, Founder and CIO, Bastion Asset Management; Gareth Shepherd, Co-Head, Voya Machine Intelligence and Portfolio Manager, Voya Investment Management; and Ankush Jain, CIO and Co-founder, Aaro Capital on the 13th of October 2022.
Download your free copy of the agenda by clicking here.