Quantitative Credit Analysis: Demystifying the World of Quant Credit
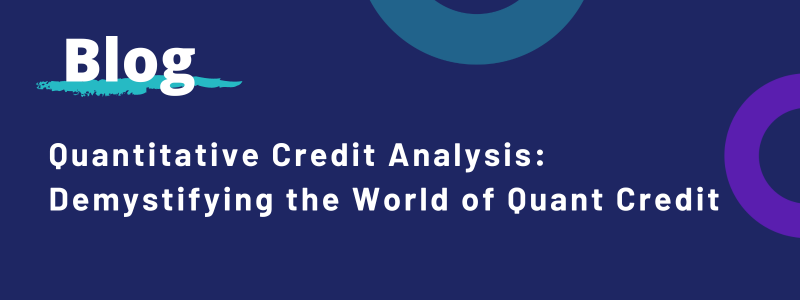
The world of finance is constantly evolving, and one of the most exciting developments in recent years has been the rise of quantitative credit analysis, often referred to as "quant credit." This approach to credit analysis leverages advanced mathematical models and data analytics to assess credit risk and make investment decisions. In this blog, we'll delve into the fascinating world of quant credit, exploring what it is, how it works, and why it's gaining prominence in the financial industry.
Don't miss new reports! Sign up for Quant Strats Newsletter
Understanding Quant Credit
Quantitative credit analysis combines the principles of quantitative finance with traditional credit analysis techniques. The goal is to create a more systematic and data-driven approach to evaluating credit risk in fixed-income securities, such as bonds and loans. Instead of relying solely on qualitative factors and expert judgment, quant credit utilizes mathematical models and statistical techniques to make credit assessments.
Key Components of Quant Credit
- Data Analytics: At the core of quant credit is the analysis of vast amounts of data. This data can include financial statements, economic indicators, market data, and more. Advanced analytics tools are used to identify patterns, correlations, and trends within this data.
- Machine Learning: Machine learning algorithms are employed to build predictive models that assess the likelihood of credit default or downgrade. These models can take into account a wide range of variables, both quantitative and qualitative.
- Risk Factors: Quantitative analysts consider a multitude of risk factors, such as interest rate risk, market risk, and credit-specific risk. These factors are quantified and integrated into the overall credit assessment.
- Credit Scoring: Just like individuals have credit scores, companies and issuers also have credit scores assigned to them by quant credit models. These scores help investors quickly assess creditworthiness.
Why Quant Credit Matters
- Enhanced Accuracy: Quantitative credit analysis is designed to reduce the subjectivity inherent in traditional credit analysis. By relying on data-driven models, it can provide more accurate and consistent credit assessments.
- Portfolio Management: Asset managers and investment professionals use quant credit models to construct and manage credit portfolios. These models help them make informed decisions about which securities to buy, hold, or sell.
- Risk Mitigation: Quant credit can help identify potential credit events and market movements in advance, allowing investors to take proactive steps to mitigate risk.
- Speed and Efficiency: In today's fast-paced financial markets, speed matters. Quant credit models can rapidly analyze a large number of securities, enabling quick decision-making.
Challenges in Quant Credit
While quant credit offers numerous benefits, it also presents challenges:
- Data Quality: The accuracy and quality of input data are critical for model performance. Garbage in, garbage out.
- Model Complexity: Complex models can be difficult to interpret and may suffer from overfitting if not carefully designed and validated.
- Market Dynamics: Financial markets are constantly changing, and quant credit models must adapt to evolving conditions.
Conclusion
Quantitative credit analysis represents a significant advancement in the field of finance. By harnessing the power of data analytics and machine learning, it provides investors and financial professionals with powerful tools to assess credit risk and make informed decisions. While it's not without challenges, the potential for enhanced accuracy, risk mitigation, and portfolio management make quant credit an essential component of modern finance. As technology continues to evolve, we can expect quant credit to play an even more prominent role in the financial industry.