Retraining ML models post-pandemic
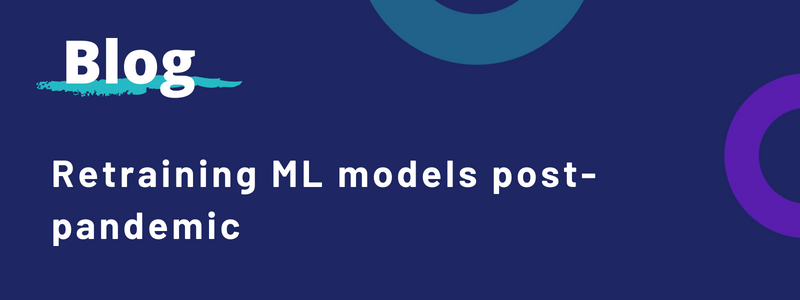
In a recent Bank of England survey, around 35% of banks reported a negative impact on Machine Learning (ML) model performance because of the pandemic.
Across the finance sector, an industry which relies on understanding and mitigating risk, COVID-19 was an unprecedented shock to the system.
The health crisis drove a major downturn that just could not have been forecasted based on economic data alone or historical predictors.
Don't miss new reports! Sign up for Quant Strats Newsletter
Financial data provider Refinitiv reported that 72% of investors were hurt by the pandemic. Some 12% declared their models obsolete and 15% were building new ones.
“Many institutions would have had to revisit a large portion of the models that they had to make them cope with what has been extreme market events,” explains Amanda West, global head of Refinitiv Labs at Refinitiv. “COVID-19 presented a large shift in many of the market dynamics.”
The crisis laid bare the Achilles’ heel of machine learning, whose effectiveness is founded on the principle that patterns and behaviours from the past will likely repeat in the future.
Algorithmic models expose these patterns in data and draw on them to predict what will happen. But when things don’t perform to pattern, their predictive powers are weakened.
Thanks to today’s abundance of available data and computing capacity, Artificial Intelligence (AI) techniques are being increasingly deployed in finance. Its application is being applied to areas from asset management to algorithmic trading to credit underwriting to blockchain-based finance.
Machine learning (ML) models use big data to learn and improve predictability and performance automatically through experience and data, without being programmed to do so by humans.
The problem is that ML models can perform poorly when applied to a situation they have not encountered before in the training data.
This was telling in the context of the pandemic. The underlying data often changed (data drift) or the statistical properties of the data altered (concept drift).
“It is a mistake to assume you can set up an AI system and walk away,” says Rajeev Sharma, global vice president at digital and technology services company, Pactera Edge. “AI is a living, breathing engine.”
For many quants, the volatility meant admitting the need for more human intervention Plus, acceptance that even with the best backtesting, there will be moments when certain strategies fail and the future will not look like the past.
If the pandemic proved anything it was that the role of human judgement remains critical at all stages of AI deployment, from input of datasets to evaluation of model outputs. Fail to do this and you drive the risk of interpreting meaningless correlations observed from patterns in activity as causal relationships.
Automated control mechanisms or ‘kill switches’ should also be used as a line of defence to quickly shut down AI-based systems when they cease to function according to the intended purpose.
Antonio Fernandez, author at Datascience.aero, says that supervised machine learning models need to be ‘supported’ in black swan events. Alternative techniques are needed too to discover new underlying patterns that help models to better understand the types of anomalies the pandemic provoked.
As the pandemic proved, agility is key to everything, even AI/ML.
And a clear learning from the health crisis was this: most of what we call artificial intelligence is a lot more artificial than it is intelligent.