Iro Tasitsiomi: One of the biggest challenges will be around Generative AI and its adoption
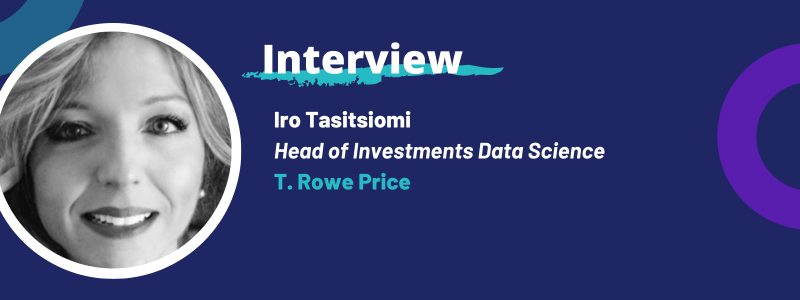
Iro will be set to speak at Quant Strats 2024 at 10.30 KEYNOTE PANEL: The quest for novel data in a saturated market. The panel discussion will take place on March 12 at the Quorum by Convene, New York.
Please give us a little introduction on your current role and what you do.
I am the Head of Investments Data Science at T. Rowe Price, an active asset manager with over a trillion in assets under management. I joined a couple of years ago after spending time in passive asset management (BlackRock) as well as the sell side (Goldman Sachs), and the world of fintech start-ups.
In this capacity I am heading the effort of augmenting our unique fundamental investing expertise with new signals, methods and approaches that can lead to new profitable strategies and products such as, e.g., around thematic investing.
Furthermore, inspired by the Generative AI potential, I am leading Research.AI, our initiative to re-imagine the way our investors are conducting research. I also lead the technology innovation workstream established by our Generative AI Steering Committee acting as the firm’s SME, driving the formulation of a comprehensive strategy for its secure adoption, as well as best implementation of buy and build solutions across the enterprise.
Last but not least, I am responsible for the professional well-being and growth of the team of wonderful data scientists that I manage.
What do you consider your biggest professional achievement to date?
The very fact that I am being asked such a question is what I consider my most significant professional achievement (if not personal, as well). As I frequently say, “I was not supposed to be here”, at least not based on the initial conditions of my life. Being the second child (and only girl) of two mid-grade school level educated parents in a small village of a few thousands of people in the mountains of Northwestern Greece neither supports nor inspires the pursuit of a career… across the world, in math and (astro)physics, quantitative finance, data science and AI…
What do you think are the biggest challenges facing data scientists/AI experts/quantitative practitioners/portfolio managers for 2024 and beyond?
I think one of the biggest challenges on the horizon will be around Generative AI and its adoption: what is it good for? Will it displace existing functions currently performed by humans? What are the legal liabilities that could be incurred through its utilization? How do we address ethical concerns, bias, private information and data security? How can we stay abreast of and compliant with the evolving regulatory landscapes?
Additionally, pivotal decisions will revolve around the "build versus buy" question: contemplating whether the technology should be developed in-house or procured externally. Will Generative AI become a standardized, commoditized offering or will there still be opportunities for differentiation through in-house development of certain Gen AI components/use cases? And more generally, the overarching matter of strategic investment allocation to Gen AI initiatives will be pivotal in navigating this intricate landscape effectively.
Navigating these challenges requires a combination of technical expertise, adaptability and ongoing learning, as well as strategic leadership thinking tailored to the needs of a company: focusing on the right solution for the right problem will be crucial in not following the herd down the wrong path.
How do data, quantitative and investment functions work together? And how could this relationship be improved?
The way these different disciplines work together depends on several factors, with one of the most important being the nature of the organization and its investment philosophy. For example, traditionally Investments Banks have been faster to adopt data and quantitative approaches in investing. Asset managers, presumably because of their higher risk aversion (?), have been slower and, maybe even to some extent, reluctant to have all these functions and have them work together.
Furthermore, when it comes to asset managers, the passive or active investment philosophy can make a big difference. For example, in active management there are clear incentives for investors to be open to data and quantitative approaches. Beating the market is the objective and signals that can act as differentiators from the rest of the market are highly desirable. In passive management, in some sense the objective is to be not differentiated at all with respect to the market.
Regardless of the setting, the way for these functions to work better is by enabling interaction and collaboration, knowledge transfer, understanding and empathy for functions involved.
Are there any particular asset classes or geographies where you see quant being applied over the next 6 – 12 months?
As data accessibility and quality continue to improve within asset classes such as Fixed Income and in markets beyond the well-established, efficient ones, it is likely that we will witness a continued surge in quantitative investment endeavors within Fixed Income and Emerging Markets.
With Gen.Ai being the word on everyone's lips and vigorous debate about the impact it has and will have on financial markets – do you think that it will seismically change the landscape or will it just automate the ‘boring stuff’?
It will probably do both. Often, the same capability can offer automation of the boring stuff or change the game all together, depending on where and how it is applied. Take content consumption or creation, for example, in business operation related use cases. Several of these tasks are overlapping and/or are repetitive, so automating these is probably the low hanging fruit.
However, when we are talking, e.g., about investment research, content consumption via LLMs can change the landscape: once upon a time she who could go physically at the parking lot of the brick & mortar business she was covering would have the information ‘asymmetry’ edge by counting cars to infer foot traffic/ how sales are going. Nowadays, information asymmetry takes a whole new meaning: data is accessible effectively by all. The edge comes from being able to locate in the vast amounts of available content, process and act on information before/better than others.
Also, I think we have been a bit narrow in our conversations about Generative AI: this past year we have been treating it as synonymous to Large Language Models (LLMs). LLMs are only one flavor of Gen AI – granted the most appealing to all users, regardless of background (hence the explosion that happened in 2023). But I think there is potential in other Gen AI as well.
At Quant Strats, we always discuss the challenges and opportunities of blending quant and fundamental strategies, and this is always a popular topic – why do you think this is?
Because combining the depth and reasoning of fundamental analysis with the rigor and power of quantitative finance offers substantial opportunities. For this to happen successfully, quants and fundamental investors must work closely together to understand each other’s approaches and discover how they can combine them in a synergistic way, thereby generating results that exceed the combined capabilities of each approach in isolation.