Judith Gu: The source of alpha is stock’s idiosyncratic risk or market dislocation
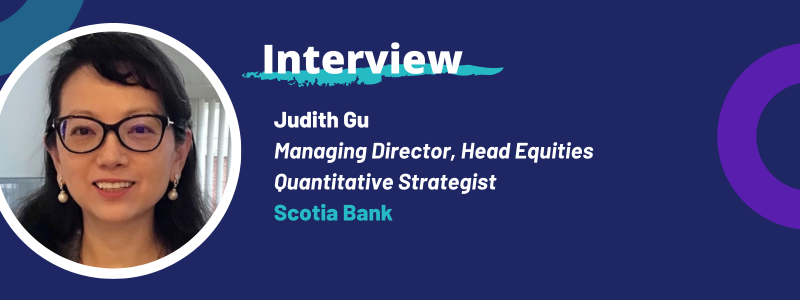
Judith will be set to speak at Quant Strats 2024 at 3.30pm PRESENTATION: An introduction to causal inference, its uses, and its applications in real-time trading. The panel discussion will take place on March 12 at the Quorum by Convene, New York.
Please give us a little introduction on your current role and what you do? What do you consider your biggest professional achievement to date?
I run quant equities for both US and Canada at Scotiabank. Our small and efficient team built a highly robust and fully automated systematic market-making, real-time risk management system within a very short timeframe through leveraging the best real-time technologies and time-proven risk management data/models. The system is serving on-demand liquidity requirements for North America clients with pricing transparency and operational efficiency.
What do you think are the biggest challenges facing data scientists/AI experts/quantitative practitioners/portfolio managers for 2023 and beyond?
The biggest challenge for quant / AI / ML/data science practitioners is no different from what it always has been – how do we trust our model/process results? Systematic trading is fast and furious, how do we ensure our models and signals have achieved risk optimal? We are integrating reasoning through causal inference into model building and quantify uncertainties through techniques like conformal prediction.
With some semblance of stability returning to the US and European markets – where do you think the next source of Alpha is?
To market maker, the source of alpha is stock’s idiosyncratic risk or market dislocation, which is our current focused R&D area.
Text data seems to be an area where firms are focusing, are there particular risks to this and how would you go about extracting the most value?
I think I can extend this question to how to integrate LLM into an equities quant trading system. It’s still at a early, whiteboard drawing stage for us. But it is quite clear that LLM is good at natural language processing but not time series predictions. LLM’s ability to parse and connect natural language makes a great tool to draw the causal graph of dependencies and feature set, and then feed this causal graph to the machine learning prediction that understands temporal structure of time series data.