Michael Oliver Weinberg: Gen.AI will both seismically change the landscape and automate the boring stuff
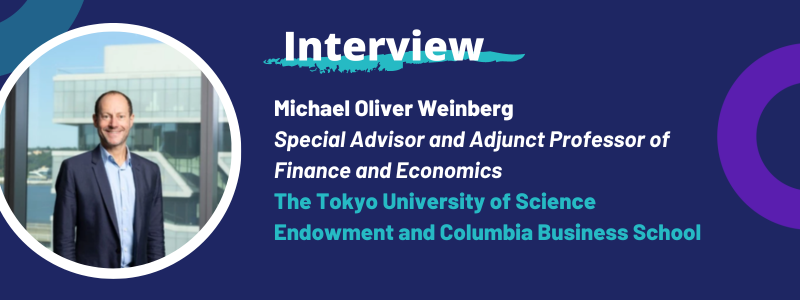
Michael will be set to speak at Quant Strats 2024 at 1.30 PANEL: Making external data sets usable, readable, and actionable. The panel discussion will take place on March 12 at the Quorum by Convene, New York.
Please give us a little introduction to your current role and what you do
I am a Special Advisor to The Tokyo University of Science Endowment, and an Adjunct Professor of Finance and Economics at Columbia Business School.
What do you consider your biggest professional achievement to date?
Managing, leading, and mentoring the next generation of asset managers and owners. It's extraordinarily rewarding to see them thriving, leading teams and businesses throughout the globe, including in Europe and Asia.
What do you think are the biggest challenges facing data scientists/AI experts/quantitative practitioners/portfolio managers for 2023 and beyond?One of the biggest challenges facing quantitative practitioners now is that LLMs are largely hyped, but the trick will be determining how to extract value from them. At one level, they are doing things that previously took immense expertise and time. On another level, they can’t do basic math or put together an accurate CV despite the very information being very publicly available on the internet. Instead, they may hallucinate, and create a fake/erroneous biography. One therefore needs to figure out a way to exploit this immensely powerful but still nascent technology.
For portfolio managers two of the biggest challenges are that information has been democratized over the past 25 years, and alpha decay has accelerated. The first challenge that portfolio managers face is two factors that have democratized information. One, Reg FD, that companies must disclose all material information at the same time to all investors. Two, the internet, which has democratized so much information that was previously scarce and an alpha source. Later we will discuss our view on information inefficiency, which we do believe is still exploitable, but the low-hanging fruit is long gone. The second challenge that portfolio managers face is that alpha decay has accelerated. Because there is such access to computing and information, alpha sources that previously took years to decay, now have vastly shorter half-lives. It’s akin to being on a treadmill that is accelerating rather than static or even easier, slowing down. One must run harder to maintain the same alpha or excess returns.
With some semblance of stability returning to the US and European markets – where do you think the next source of Alpha is?
Asia is the next source of alpha. There are 35 equity markets in Asia compared to only 27 in Europe. In addition, the market cap of Asia is just over 20% of the world versus roughly half of that for Europe. Asian markets have historically had significant retail participation, which due to behavioral psychology often creates alpha and inefficiencies that quantitative managers can exploit. Keiretsus in Japan, and Chae-bols, in Korea, which are effectively conglomerates with cross-shareholdings similarly create inefficiencies that provide alpha. There is a multitude of legitimate possible ways to attain a research ‘edge’ in Asia as well, including with alternative data, whether corporate exhaust, scraped, or from governmental agencies.
What is the biggest potential economic banana skin that could shock the system?
The biggest potential economic banana peel would be another hit to banks' balance sheets. Akin to what happened in March 2023, but this time a credit crisis rather than a duration crisis. In March of 2023, we had write-downs due to the Fed raising rates, and the duration mismatch due to our fractional banking system model of borrowing short and lending long. Consequently, equity was diminished due to the inherent duration mismatch in the model and system. However, this largely had nothing to do with the credit quality of the loans. As SOFR has gone from 0-1% to 5% over the past year, if there were material write-downs on the bank's real estate holdings, due to lower occupancy, lower net operating income (NOI), higher interest rates, and consequently higher capitalization rates, which imply lower values, this would impair the value of bank’s loans. This could impair the bank's equity and market valuations. In turn, this necessitates bolstering their capital ratios via equity or other capital injections.
How do data, quantitative and investment functions work together? And how could this relationship be improved?
Like in a great opera, where the libretto, the score, and the mis en scene all need to work together, in a systematic fund, similarly, the data, coding, and investment philosophy must all be in harmony. In our experience there is effectively a Venn Diagram for systematic investors with three circles: 1) Coding, 2) Data Science, and 3) Investment acumen. It's very easy to find those that excel in one of those attributes. There are still a considerable number who excel in two of the attributes, typically Coding and Data Science. But to find those that excel in all three, particularly coder/data scientists who understand investing is far less common.
In fact, over the past decade, we have seen countless Silicon Valley success stories gravitate towards systematic strategies only to be humbled by how much harder it is to trade financial markets. There are many reasons, including the non-stationarity of markets, i.e. coding a system to recognize a cat, when a cat looks the same today as over a century ago in Victorian England, is not challenging. However, markets are non-stationary, and constantly changing. Sources of alpha are arbitraged away and become beta.
Remember the first generation of quant funds that built successful and profitable business based on buying cheap companies? And hedge funds that shorted expensive ones? Now that is available for no-fee via ETF and index funds. Alpha became beta. There is little if any excess return or value from that, whereas years ago it was immense.
An example, where an asset owner might determine that the data, quantitative systems, and investment functions are not working together is if the expected trade duration does not align with the profitability over a similar duration. For example, if the trade duration is short-term, then one should expect to be profitable over similarly short periods. If over a long period this strategy is not profitable, this would be a red flag, as it should have been profitable over short(er) periods. The opposite also holds. If one is trading, with the expectation of profit over months or years, one should not expect consistent intra-day or daily profit. Gets back to the opera analogy we started with, it all must be in harmony.
Are there any particular asset classes or geographies where you see quant being applied over the next 6-12 months?
Increasingly over time, quantitative methods will be applied to areas where it hasn’t been historically. Private equity is one such example. We could see a world where eventually private equity employs quant from sourcing through exit.
We already know a private equity firm that uses quantitative methods to monitor and screen companies, then write letters to prospects over time, to in turn generate interest from management to divest stakes. The letters are so well written by the machine the private company owners don’t know they are machine-written and assume they are generated by humans.
Then one could use systematic methods, NLP, and LLMs to write contracts, which could even be Ethereum ‘smart contracts.’
We know another PE firm that is using NLP and LLMs to generate discussion topics and questions for monitoring their portfolio companies. Similarly, quantitative methods could be used to monitor and flag if companies are in line, above or below Key Performance Indicators (KPIs).
Then one could even use systematic methods to compare the valuation and performance of the companies to other public and private companies over history to flag potential optimal divestiture timing.
The above is effectively a start-to-finish quantitative PE system. Over time human plus machine with a strategy like this will be superior to what is largely today’s human-only PE strategy. And though we are seeing fragments of it applied by various firms, we are not seeing any firm embrace the entire concept. This is not surprising. In fact, we would expect to see this from a disruptive, new entrant. Just as Tesla disrupted auto and rocket manufacturing, or Amazon disrupted retail.
With Gen.Ai being the word on everyone's lips and vigorous debate about the impact it has and will have on financial markets – do you think that it will seismically change the landscape or will it just automate the ‘boring stuff’
Gen.Ai will both seismically change the landscape and automate the ‘boring stuff.’ As we just discussed, our forecast for how it may impact PE investing includes both aspects. Ranging from revolutionizing the entire investment process via automation and data to automating the boring stuff, i.e. prepping for meetings, writing contracts, writing sourcing emails, and monitoring KPIs.
The data market seems to be incredibly saturated at present, with more data being available than at any other time in human history – do novel data sources still exist?
As Alphonse Karr, the French novelist stated in 1849, Plus ca change, plus c’est la meme chose, which is, the more things change the more things stay the same. The commercial data market is incredibly saturated. For years we have been asking, which top quant fund doesn’t have satellite or credit card data? That has been commoditized for years. What big quant fund can’t afford that or doesn’t have it?
Where the inefficiency still continues to lie is in more creative data sourcing and ensembling, but we will talk about that later. Eighty percent of the world’s data will have been created in the past couple of years, and like Moore’s law, this will continue to be the case. The opportunity therefore is not in structured financial data, like Bloomberg or credit card data. It is in unstructured financial data and structured and unstructured non-financial data. There is an immense amount of private company data, governmental (non-financial) data, Freedom-of-Information-Act (FoIA) corporate exhaust data, internet of thing data, and now textual data which can more easily be structured with the accelerated advent of Large Language Models (LLMs). This is all ripe for picking and alpha extraction.
Is the value now in merging different data sets together to give a ‘full picture’? And what challenges does this bring?
Yes – we alluded to that in the prior question. Though we do believe much data is commoditized where there can be incremental value is by ensembling this data, ideally with more valuable, scarce data sets. To use a machine learning analog, think of it as boosting, taking a bunch of weak algorithms or learners and ensembling them to create a stronger one, but this time applied to data, as opposed to algos.
The Credit market seems to be an area of interest in recent times, what has spearheaded this growth in interest, and can quant be applied there effectively?
Over the years, where we have seen systematic investors excel in credit is where they have a proprietary data set. Unlike in public equities, which are more typically exchange-traded, with large companies offering access for fees, credit markets are often non-exchange traded. Therefore there are opportunities to create these bespoke data sets, which in turn become very valuable. Some years ago, we first saw a municipal bond manager create a bespoke data set that it used to apply rudimentary machine learning techniques.
More recently, one of the systematic credit manages we have immense respect for has spent the last few years creating just such a data set. He is generating immense alpha from it and materially outperforming both indices and comparable discretionary managers. But it took significant investment, time, data science, and meticulousness for him to create this. Over time, this will be like any inefficiency and more competition will arbitrage it away, but for now there is still much lower-hanging fruit than in equities.
Text data seems to be an area where firms are focusing, are there particular risks to this and how would you go about extracting the most value?
The risk to using textual data is that if one is applying one's own client or proprietary data, that one is not populating LLMs with that, and in turn inadvertently publicly disseminating it to the world, including competitors. This is already coming under greater regulatory scrutiny by the Securities Exchange Commission (the SEC). For this reason, many financial institutions, including ones we have worked for, preclude their employees from using public LLMs. That said, the solution is simple, having ones firms data lake paired with a bespoke enterprise version of the LLM. Obviously then one has to be comfortable with the security of that, but that is not insurmountable, just as the use of the cloud has become accepted and in fact pervasive.
Is the AI winter coming to an end? And can quant be applied to the digital asset space or is there too much regulatory divergence?
We would say it was more like we were recently in a crypto Fall, which with the advent of LLMs, accelerated it past the Winter and right into Spring or Summer. There have been countless crypto winters over the past 70-plus years since machine learning was invented by Turing and others post-World War II. We were already making inconceivable strides in machine learning over the past few years due to the confluence of 4 factors: 1) record low processing storage costs, 2) record data growth, 3) the advent of data science and 4) machine learning working, somewhat reflexively due to the prior 3 factors. However, the advent of LLMs just took it to a new level. That said, it in turn sets itself up for the next AI winter because the level of hype is so strong that it may once again disappoint. We are seeing this in valuations of AI start-ups, which we would not be a buyer of today at these valuations, having been a successful portfolio manager at Soros, through the first ‘tech bubble’ in the late 1990’s.
Are you seeing quant investing being used in new geographies/ asset classes /where are you expecting some interesting quant stories to emerge from?
The new quant frontiers are primarily credit, private equity, and in Asia.
At Quant Strats, we always discuss the challenges and opportunities of blending quant and fundamental strategies and this is always a popular topic – why do you think this is? What do you think are the most important questions for quants when?
Well, typically the blend is a function of discretionary managers who are inherently not quants, but either because; 1) they realize their alpha is, has, or will be picked off or 2) they believe their clients want them to be quantitative or more quantitative, they morph into ‘quantamental.’ For these reasons we believe the winning solution will be human plus machine, but more of a fully systematic solution. We do not believe the winning solution will be quantamental, or a ‘bastardized’ quasi-quantitative strategy, which is really a closet discretionary manager with a quant overlay.
Don't miss Michael's panel at Quant Strats!
Subscribe to Our Free Newsletter