Petter Kolm: The holy grail is to use the data you have in novel ways
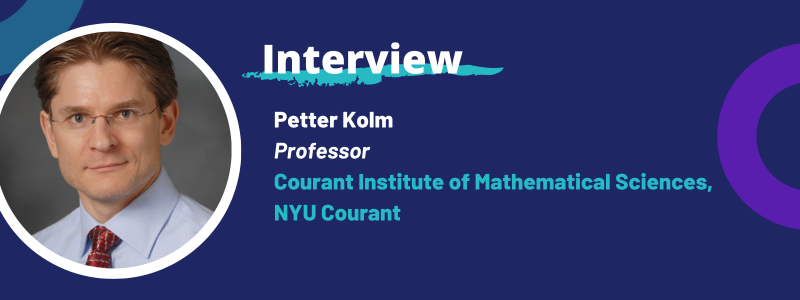
Petter will be set to speak at Quant Strats 2024 at 1.50 pm on the PANEL: Where can ML realistically outperform humans? The panel discussion will take place on March 12 at the Quorum by Convene, New York.
Please give us a little introduction on your current role and what you do
Petter Kolm is a Professor at NYU Courant. In 2021, he received the "Quant of the Year" award from Portfolio Management Research (PMR) and the Journal of Portfolio Management (JPM) for his significant contributions to the field of quantitative portfolio theory. As a consultant and expert witness, Petter offers his expertise in areas such as machine learning, portfolio management, risk management, and trading. He has co-authored numerous articles and books on quantitative finance and financial data science. Petter serves on several company advisory boards (including Aisot Technologies in Zurich and GoQuant in Miami), editorial boards for academic journals, and boards of directors for professional associations. Petter holds a Ph.D. in Mathematics from Yale, an M.Phil. in Applied Mathematics from the Royal Institute of Technology, and an M.S. in Mathematics from ETH Zurich.
What do you think are the biggest challenges facing data scientists/AI experts/quantitative practitioners/portfolio managers for 2023 and beyond?
Major strides have been made in financial ML in the last few years. However, most of these developments are not broadly known. This puts companies and PM who deploy these techniques at a clear advantage. There is a clear first movers advantage here, especially on the alpha side of the business.
The data market seems to be incredibly saturated at present, with more data being available than any other time in human history – do novel data sources still exist?
As novel data is becoming harder to find, the holy grail is to use the data you have in novel ways. Perhaps surprisingly, there are often big wins to be had by better processing and labelling your data. Merging different data sources can be challenging, but often results in significantly improved signals.
The Credit market seems to be an area of interest in recent times, what has spearheaded this growth in interest and can quant be applied there effectively?
Electronic trading in corporate bonds is booming. Nobody would have believed ten year ago that would happened. In this area, there is big demand for building models for risk and return forecasting, market making, execution and TCA. To a large extent, and perhaps not so surprising, many of the recent developments in this area mirror algos and models in equities.
Text data seems to be an area where firms are focusing, are there particular risks to this and how would you go about extracting the most value?
Large language models (LLMs) have taken world by storm. And it is not surprising. Why? Because they are in big ways changing the way we work, communicate, code and invest. Integrating LLMs in your workflows increase productive and decrease turnaround time. On the trading and investing side, LLMs providing many interesting opportunities, some of which we are exploring in research right now.