Speaker Interview: Michael Rothenberg, Global UDS BDM, FinServ, Energy, & AI at Dell
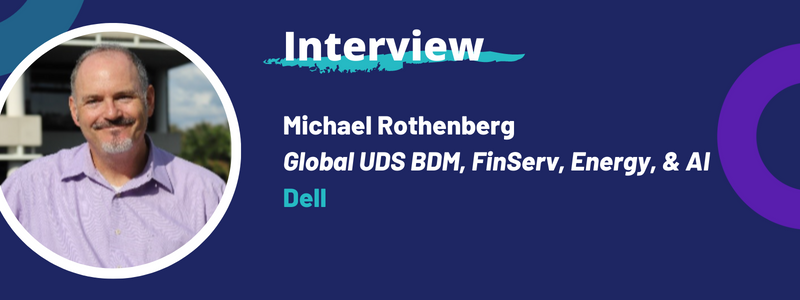
1. Please give us a little introduction on your current role and what you do.
My official title is Global Business Development Manager, but the role is more than that. I have 3 areas that I work on. First, I’m providing SME support to the teams in the field and directly to customers. My areas of expertise are Data Analytics/AI, Financial Trading/Quantitative trading, Utilities, Oil & Gas. Anyone with questions in those areas be they Dell, partner or customer can call upon me for answers. Second, I work with SW and channel partners focused in those areas of expertise to build go to market strategies, product testing, validated designs/architectures, events, etc. to help drive market awareness and knowledge of our solution offerings. Lastly, I work on content and collateral creation the help train our sales and pre-sales teams on how to help our customers with their challenges and provide solutions on Dell and partner products.
Don't miss new reports! Sign up for Quant Strats Newsletter
2. What do you consider your biggest professional achievement to date?
I’ve only been at Dell a little over a year so my largest professional achievement, so far, came at NextEra. Building out the smart meter infrastructure, data collection, and models that analysed the data from over 6 million smart meters was a lot of fun with a lot of challenges. The modelling of multiple ISOs (energy markets) feeding into a successful trade recommendation engine for cross border wheeling of power would be another one. Many hurdles needed to be overcome for that algo to be profitable. Working on an energy trade floor is similar but also very different than a financial one. The differences in features that influence the predictive outcomes require different outlooks and approaches.
3. What has been your /your firm’s top 3 priorities for the coming year?
For Dell Unstructured Data Solutions, the coming year is going to be focused on providing whole solutions to our customers, cutting edge technology improvements (Billions in R&D), and world class customer service. For the last few years, Dell has pivoted away from being just a HW vendor and is focusing on delivering an end to end solution for the customer. We are one of the few companies who can actually build and deliver the whole stack from storage, through compute and networking. In conjunction with our large domain of SW partners, a full solution can be delivered to our customer regardless of industry or verical.
4. What do you think are the biggest challenges facing data scientists/AI experts/quantitative practitioners in 2023 and beyond?
The largest challenge I see for the practitioners in the field remains the issue of the ‘last mile’. Once a model has been built, tested, and approved for production getting into production and feeding it the correct data sets in a timely manner is a very challenging task. There are two factors here that make this challenging: Data growth and very different environment requirements between R&D and production.
The data universe that practitioners are using in their models is expanding along side of the amount of data covering existing variables. Practitioners will pull data from anywhere they deem necessary with the expectation it may improve model results. Looking for that edge is their job and this is a good thing. However, this This data is sometimes very disparate and found in a variety of places. Both of these factors contribute to the complexity of the problem by making it difficult for the data delivery team to deliver the data to the model when it is time for production.
The 2nd factor is a basic environment challenge. For R&D you want fast wide open data, not necessarily clean, and easy access that encourages exploration and use. The compute needs to be high performant (lots of iterations), flexible and expecting an ad hoc job stream. The individuals are typically very high achievers looking to learn, move fast, and willing to experiment with bleeding edge algorithms. The production environment is different in that we want strict access and governance with well defined, clean data and data lineage for auditability. The compute has to be scalable with high availability, easy to manage, and low latency. The crew running production has goals of sustaining operations at lowest cost while optimizing reliability and unintended changes. These two worlds can sometimes be at odds and those that build the infrastructure need to serve both.
5. Market and political uncertainty over the last year has seen unpredictable outcomes for some quant firms – how do you think quant firms can prepare for increased uncertainty to come and manage the 40-year inflation high that was seen in 2022?
The economic environment right now is incredibly challenging! Firms are announcing record profits and then layoffs in the same week. Inflation is wild, yet the labour market and spending is still hot. The fundamental economic signals that would fill most feature sets are all over the place right now. Building and trusting a model in this time is difficult as any back testing will be suspect given the extreme circumstances of the last few years.
Preparation should continue along the lines of being more conservative in some areas and more aggressive in others. Understanding that back testing results over the last few years could be suspect and that the results should be looked at in a more conservative manner is an example where to be conservative. Understand that the risk levels may be greater than you perceive due to conflicting economic signals. However… no risk no reward! In times like these, a good call can turn into a great call as everyone tightens up and uncertainty freezes actions. Being ready to act when such opportunities present themselves is key.
Still, not much has fundamentally changed… understand the risks, mitigate them the best you can, make your decisions with the best information you have at the time, and go for it!
6. To what extent do you see the use of blockchain/crypto integrating into capital markets? As crypto is becoming more mainstream, how have hedge funds responded and what could be the potential impact on capital markets?
a. What are your predictions for quant investing in crypto?
I really like crypto and blockchain. I have been involved for the last 8-9 years at various aspects of the chain from building out a small 2MW mine, to day trading, and then looking at venture investments in crypto based start-ups. It is a crazy space which makes it so exciting I suppose! Reminds me of internet start-ups in 2000. A lot of those crashed and burned, but some have transformed the way the world does business!
Blockchain as a tech has a function and place in capital markets. I have no doubt of this. However, I’m not sure we have seen the best application of it yet. Coins, currencies, or psudo-currencies are the beginning and we are just now starting to really understand some of the pitfalls and risks. As far as investing in coins, absolutely! Crypto should be a part of everyone’s portfolio from home investor to hedge funds… just make sure you understand the risk profiles!! Right now, it should be considered a very risky element and treated as such in portfolio balancing.
These are the vectors that the markets are using to get into crypto, but there is a lot more to Blockchain than just coins. I can’t wait to see where it all goes!
7. What is your advice to funds hoping to get new systematic strategies into production quickly and more often?
This goes back to the larges challenge of working with DA/AI! That last mile problem is hard to solve. There are some good DataOps & MLOps providers out there that I would recommend taking a look at, but something fundamentally has to happen 1st before even embarking on this journey. You have to have your data in order and you have to be able to put new data sources into the mix rapidly and in such a way as to be readily available to your modelling teams.
The world is changing quickly and change is accelerating. The edge in DA/AI is exactly this question: those who get their models from R&D into production faster get to market 1st and make more from it. This is accomplished only if you have a strong data management foundation that is both flexible and scalable.
8. ESG and sustainable investing is still a key topic but something that quant professionals are not always engaged with. How do you see this progressing in the coming years and influencing portfolio management?
ESG is in its infancy and as such there are a lot of things about it that are undefined. This makes it challenging to put into models as features as the impact of ESG programs is anyone’s guess right now. As regulatory efforts advance with regards to reporting and eventually limits it will be come a driving factor for some instruments. That is a good amount of time off in the future. For now, keep and eye on it, read about it in the annual reports, understand that there are investor segments that consider it important, but the data is not standardized or entirely accurate right now so I would keep it out of quantitative models until that changes.
9. Privacy and regulation surrounding the responsibility and ownership of data is still an area being discussed. What measures are you predicting will be put in place to navigate any foreseeable data privacy challenges while searching for alpha, and how can funds learn to navigate these regulations and policies?
Those that own the data own the value. Everyone wants your data even if they have no idea how they will use it. Data is the new gold, the new oil! This is true, but also is a dangerous incentive to capture, keep, and sell what is not yours.
It is hard to predict what regulations will come and more difficult where they will come from. Inconsistent data policies across the globe is an even bigger challenge than dealing with the individual policies themselves.
Regulation is coming, but as a quant I’m not sure it will have great effects on the daily job. The biggest risk will be in Alt data sources and investigating the source’s data policies will likely fall on the legal department’s task list.
10. Alternative data is still considered a source of alpha for many – what roadblocks do firms tend to come across in sourcing, cleaning, and using this data? How do you view the alt data market at present?
The easy AI money is long gone. Alt data sources should be included in looking at features that help explain market activity. I do not think that this can be avoided. Alt data sources are certainly becoming market movers; just look at the GameStop events. How we use that Alt data in our models is where the skill comes in. Being able to extract meaningful insights from the unstructured data (tweets, posts, images, videos, articles, blogs, etc…) will be a highly valued skill in the years to come as our digital transformation and increased social/digital media use continues.
However, this data is wildly inconsistent, constantly dirty, missing values, full of junk, and all the other things that take up non-value added time from quants. The stat is variable, but something like 85% of a Data Scientists time is spent gathering, cleaning, and reformatting data into something that can be used as model input. That is with fairly benign numerical data. Alt data is on another level of disarray completely.
Entities that can offer a verified, curated and cleaned alt data set that keeps outliers and remains in consistent formats will find demand for their products. Even then there will be the question about what got removed and would that data change my model results. This is the challenge!
11. Our research this year showed a lot more firms and practitioners talking about NLP than usual – why do you think this is? Where are seeing the optimal utility for NLP and where does it have the potential to go?
NLP is getting a ton of press right now due to ChatGPT. It is certainly a major category of modelling and much can be derived from the results of NLP models. It is also one of those models that reports results that are easily understood by humans vs. other models that report out just numbers. This makes it easy for us to identify with and also be amazed by.
For me, NLP is just another tool in the kit to use when looking at solving a problem or building a solution. DA/AI modelling techniques and capabilities are booming and will continue to accelerate. There is so much innovative research being done around the space that advances are coming in leaps and bounds. It is also one of those areas where advances can be implemented right away. In the case of web enabled utilities, the entire planet can have access to it almost instantly vs. more hard science advancements that may take years to get into production.
I actually see NLP in a couple of different classic workloads. Understanding, classifying, and searching digitized documents is a big space here. Most folks do not realize just how many written contracts, documents, reports, etc are generated by businesses on a daily basis. They are filed away and the information and data contained within them is essentially lost. NLP algos can reclaim that lost gold.
Chat bots or digital assistants have been kind of clunky for a while. With the new advancements they may become much more useful. As they become indistinguishable from actual humans, the adoption and acceptance will rise also.
But it does not come for free. Large language models are expensive to train and to maintain. A previous model to ChatGPT, GPT-3, took several million $s in compute time to train it. This is not something that a small business is capable of funding on their own. Especially if the training doesn’t yield a model that is capable of providing the targeted solution and needs a re-train. NLP models can get very expensive, but also can yield amazing results.