Samuel Mugel: Data abundance will continue to be an opportunity and a challenge
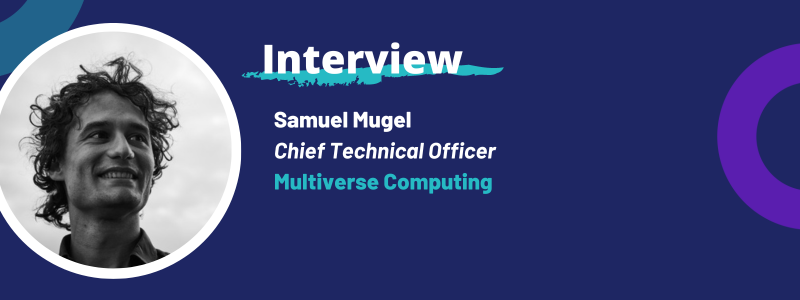
Quant Strats 2024 will take place on March 12 at the Quorum by Convene, New York.
What has been you /your firm’s top 3 priorities for the coming year?
Our top 3 priorities are:
- To transition from a services based business model to SaaS;
- To sell 100 licenses
- To release our new product for Large Language Models: CompactifAI
What do you think are the biggest challenges facing data scientists/AI experts/quantitative practitioners/portfolio managers for 2023 and beyond?
Data abundance will continue to be an opportunity and a challenge. This trend will only increase now that we have powerful tools for generating content. What’s more, synthetically generated data will tend to reinforce the patterns that generated it in training new models, making realistic training and the prevention of hallucinations more complex. This is where you really need advanced techniques like Tensor Networks to eliminate redundancy and improve model performance. This also massively reduces training costs, which are set to become prohibitively expensive by 2026.
With Gen.Ai [gen.ai] being the word on everyone's lips and vigorous debate about the impact it has and will have on financial markets – do you think that it will seismically change the landscape or will it just automate the ‘boring stuff’
The spreadsheet was a revolution in that it made organising data free. The internet made knowledge sharing free. Gen.Ai is making content creation free. Even more important for financial markets, it makes vectorizing content free, meaning you can now gain insights from data we used to need a human to interpret. This is important because (1) a machine can sieve through massive volumes of data and act on insights and correlations real time, meaning there’s a significant speed advantage; and (2) Large Language Models can assist humans in interpreting complex data, bringing untrained humans’ performance almost to the level of experts for many tasks, which has a profound impact on talent abundance.
Text data seems to be an area where firms are focusing, are there particular risks to this and how would you go about extracting the most value?
Fast textual analysis is exciting because it opens the doors to sentiment analysis driven trading. It introduces many challenges because you need to work with live data and respond in an extremely short time-frame while maintaining query privacy. We simply don’t have the hardware to support this volume of inference. That is unless we are able to considerably speed up inference and reduce the memory footprint of Large Language Models. This is exactly what we are achieving with our product CompactifAI.
Are you seeing quant investing being used in new geographies/ asset classes /where are you expecting some interesting quant stories to be emerging from?
New methods are emerging to price asset classes which were intractable until now, like Bermudian swaptions. We’ve seen this for instance with neural networks, which far outperform traditional methods for exotic options pricing and hedging. Being able to efficiently and accurately price and calculate the Greeks for such complex asset classes will open up new markets and improve economic resiliency.