The expanding role of AI in hedge fund management
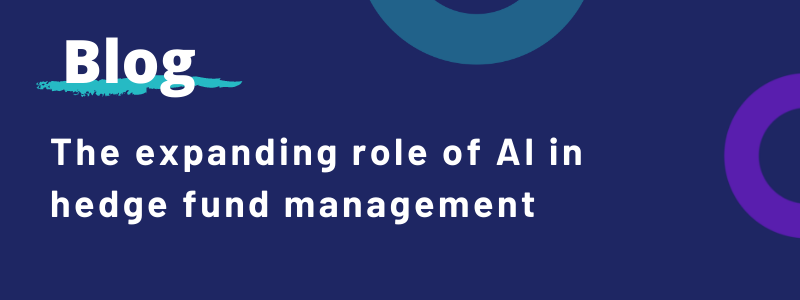
Today, hedge funds can – and do – base their investing strategies on the models and tools constructed by AI.
There are already many examples of such models using AI as a helpful tool to improve investment intelligence that are outperforming more traditional investment methods.
Perhaps the most well-known is Renaissance Technologies, one of the world's largest quant managers.
The fund, with $165 billion in AUM, was launched in 1982. It has transformed its strategy through its use of AI, often eschewing recruitment of typical Wall Street types with financial backgrounds in favour of pure data scientists.
Don't miss new reports! Sign up for Quant Strats Newsletter
Renaissance is famed for its systematic trading based on computer models and quantitative algorithms.
It attributes its success in providing investors with consistently strong returns to its investment in AI trading techniques. (Even if, lately, like many hedge funds broadly, it struggled to navigate the increased market volatility brought on by the pandemic.)
But across the board, AI is now being increasingly used to transform asset allocation, trading processes, risk management and other areas of portfolio management – as well as back-office operations.
It is allowing analysis of masses of data, the prediction of corrections in supply and demand imbalances, and the forecast of market movements for tactical asset allocation.
Quantitative and discretionary managers, for example, are tapping into AI by analysing the oceans of new data being generated by smartphones, the internet, and other innovations to extract information on retail foot traffic, satellite data, and more.
They are using it to parse massive volumes of text through natural language processing to gauge sentiment on the economy, such as employment and business activity.
Overall, AI is enabling managers to deliver portfolios with better out-of-sample (i.e. using all available data) performance for investors, while rebalancing and automatically managing risks with minimal transaction costs.
But let's take a step back. Hedge funds began as far ago as 1949 as entities designed to 'hedge' risk by alternating between short and long-term strategies, making it less likely for the investor to lose money.
The classic long/short equities model was introduced by Alfred Winslow Jones, the first money manager to combine short selling, the use of leverage and shared risk through a partnership with other investors, and a compensation system based on investment performance. Today he is seen as the 'father' of the hedge fund.
A hedge fund, essentially, is a relationship between a professional fund manager, (often known as the general partner), and investors, (sometimes known as limited partners).
Today's hedge funds are searching for outsized returns. They have the freedom to tap into any combination of broad investment strategies and to invest how they chose, including highly speculative fields, in their search for returns.
That's because hedge funds can basically invest in anything: be it land, real estate, stocks, derivatives, or currencies. (Mutual funds, on the other hand, concentrate on stocks or bonds and long-term investment goals.)
The goal of any portfolio optimiser is to honey-pick the best portfolio out of all possible portfolios, according to the investment objective.
The objective typically seeks to maximise the expected return while controlling for an acceptable level of risk.
AI is proving a useful tool in this respect for risk management and compliance. It is permitting more intensive analysis of data than in the past to identify illegal patterns and risk signals across data sets and trigger response protocols.
Modern portfolio theory measures the effects of diversification when risks are correlated and provides a framework for measuring diversification.
When it comes to portfolio optimisation, there are three principal approaches to long-term strategic asset allocation across hedge fund strategies:
· Equal weighted approach
· Equal volatility weighted approach (risk-parity)
· Mean-variance optimisation (Markowitz)
Even as far back as 2018, BarclayHedge's Hedge Fund Sentiment Survey found that over half of hedge fund respondents (56%) were using AI to inform their portfolio construction decisions, nearly triple the 20% reported in 2017.
Around two-thirds of those using AI were doing so to generate trading ideas and optimize portfolios. Over a quarter were using it to automate trade execution, according to the survey, to automatically identify the best times, size and venues for placing trades.
In 2020, Institutional Investor reported that hedge funds using AI returned almost triple the global industry average
AI-led hedge funds reportedly generated average returns of 34% in the three years to May 2020, according to the report, compared with a 12% gain for the overall global hedge fund industry in the same period.
So where will AI in hedge fund investing be a decade from now? Things are moving too fast to say. But it’s likely the gains will continue to be evolutionary.
Quants are now using AI to automate decisions that used to be made by humans. In the future, will AI automate the decisions that are made by quants?